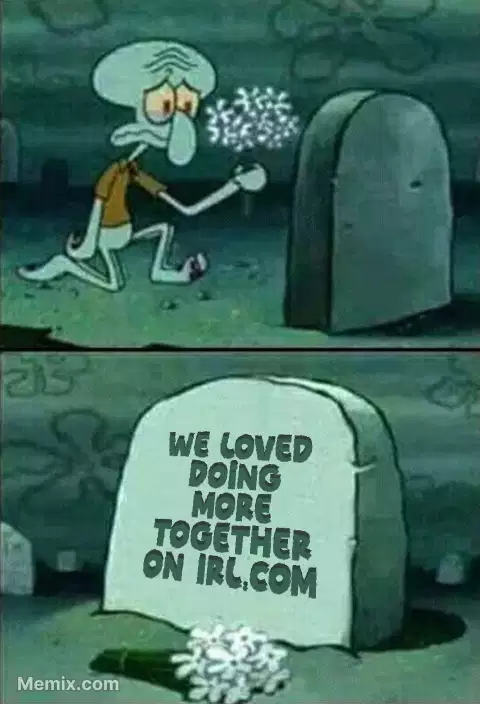
IN REAL LIFE
TM
Do more together
IRL has shut down as of
June 27th at 12pm PDT
We thank you for hosting your community chats with us, and we wish your community great conversations on other platforms moving forward.